Statistical learning models are increasingly used for decision-making in private and public organisations. This column discusses how they could be usefully applied to predict export success with firms’ financial accounts. After training algorithms on the experience of exporters and non-exporters, one can obtain out-of-sample probabilistic scores that catch the distance of a company from exporting status. The authors point to at least two cases when predicting the next exporters might be useful: trade finance and industrial policy design.
As is often the case, science fiction anticipates the future. In the 1950s, a novelist imagined a future world in which crimes could be foreseen by a combination of machines and psychically gifted individuals. Minority Report eventually became an action movie in the 2000s, and flocks of spectators were still captivated by the idea that human beings and machines could cooperate in predicting the future. Only about twenty years later, we know that policymakers are not psychically gifted, but they finally have machines that may help select policy targets.
Machine learning models have proven powerful tools for decision-makers in both public and private organisations. As in the old science fiction, we do have an algorithm that profiles potential criminals in public courts.We also have an algorithm to spot accounting fraudsters (Usuki et al. 2020). However, most of the benefits from harnessing big data and machine learning have been picked by private companies, who seized the opportunity of predictive models for a wide array of operations, from inventories management to customer relationships and, in the case of the financial industry, from credit risk management to portfolio optimisation.
Yet, we argue, there are still unexplored and unexploited benefits to come for evidence-based public policy design. For example, De Blasio et al. (2018) show how a proper machine-learning framework can target subjects that could gain more from a tax rebate. Most recently, new methods have emerged at the intersection of statistical learning and causal inference that improve ex-post policy evaluation methods (Athey and Imbens 2019), whose aim is to exploit observable information to predict policy counterfactuals with a higher accuracy.
In a recent paper (Micocci and Rungi 2023), we focus on ex-ante predictive analyses for international trade. We exploit firm-level financial information to predict whether and how close firms are to becoming successful exporters. Our general intuition relies on the long-established evidence that exporters and non-exporters are heterogeneous and, thus, statistically different (Mayer and Ottaviano 2008, Bernard et al. 2012). While trade offers general welfare gains, only a few firms may be able to sustain the costs of handling different regulatory environments, meeting different consumer tastes, and establishing marketing and logistics channels.
Therefore, our basic intuition is that machine learning algorithms could extract non-trivial information that differentiates exporters from non-exporters. If we do so, we can also measure how far a company is from becoming an exporter based on what we know about who previously succeeded in international markets. The first step is to train different algorithms on a representative sample that includes exporters and non-exporters. We select an extensive battery of 52 predictors encompassing different aspects of a firm’s economic activity (financial constraints, total factor productivity, size, age, innovation, industrial affiliation, geography, etc.) with reference to specific literature. Once we assess which algorithm predicts with a better accuracy,then we can focus on non-exporters and their ability to propose on international markets.
We can better grasp the idea by looking at Figure 1, plotting actual predictions obtained for non-exporters in France in 2010-2018.
Figure 1 Predictions for non-exporters (scores) after a Bayesian additive regression tree with missingness in attributes
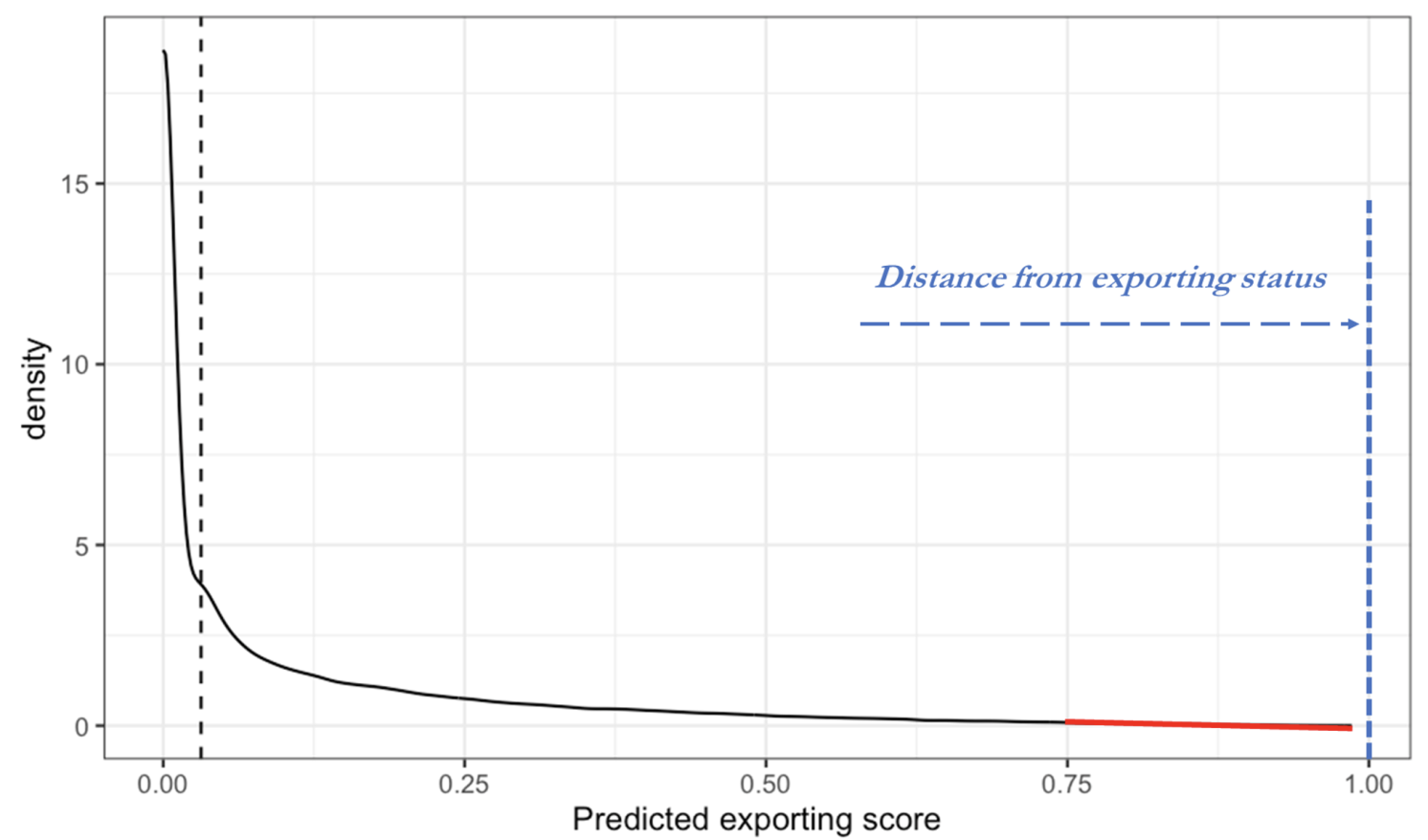
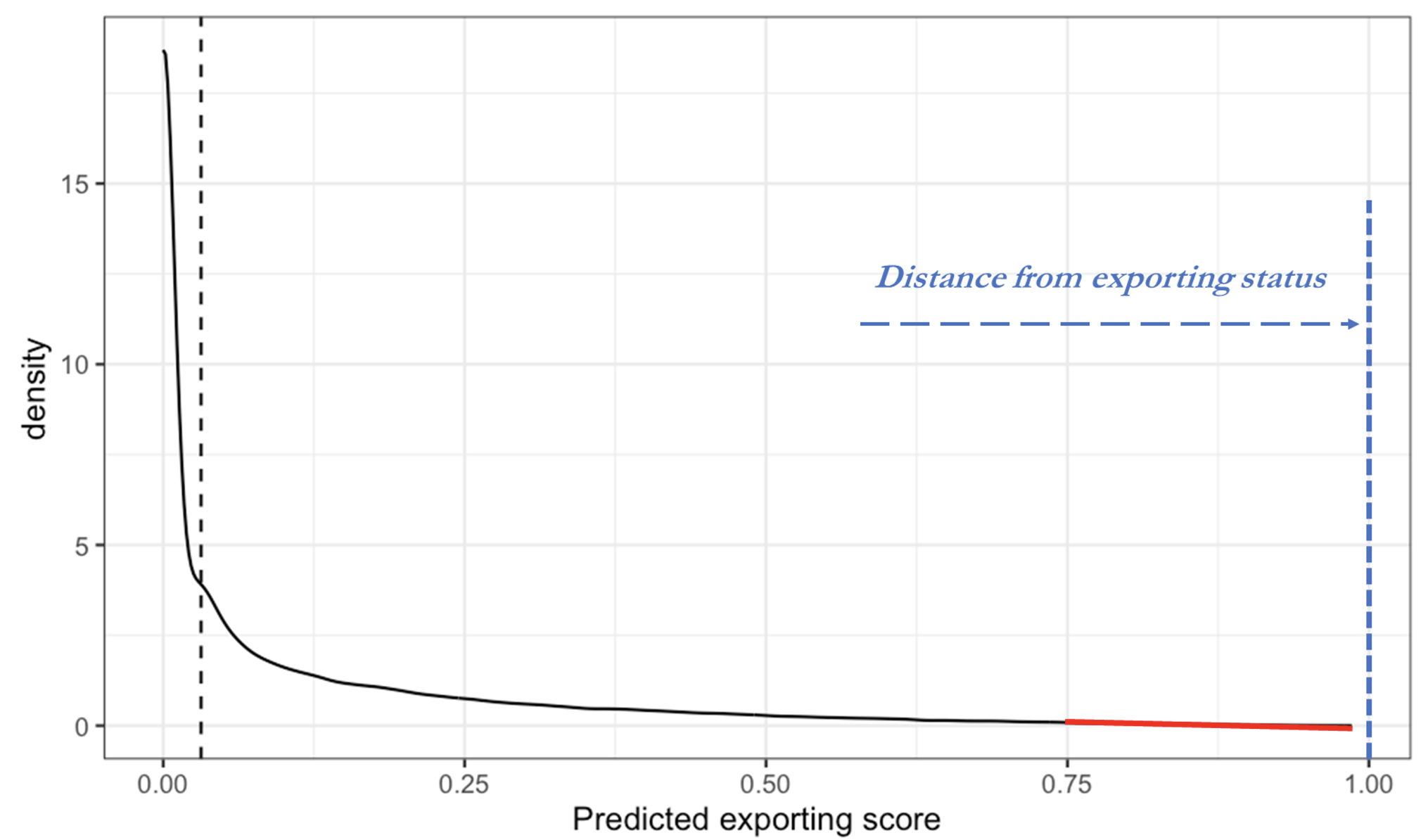
Source: Micocci and Rungi (2023)
After running our best algorithm, we can obtain a segment of predictions that goes from zero to one, where one stands for the certainty of an exporting status. By construction, the shortest the distance from one, the highest the odds that a firm can successfully become an exporter. From a broader perspective, if we look at companies located on the right tail of the predictions in Figure 1, we have information on who the next exporters can be.
But how useful is predicting the next exporters? We can think of at least two cases in which one may want to know the export potential of a company. The first is in the case of trade finance, when trade promotion agencies or private financial intermediaries decide how to allocate resources to the internationalisation of enterprises. Is an investment project worth financing? What credit risk can one attach to the applicants’ projects? Is the company able to sustain the competition after reaching international markets? A predictive score trained on the experience of previous successes and failures can help design credit policies for organisations that aim to promote internationalisation. For example, one may conclude that applicants are already compliant with all the characteristics of a successful exporter, and, in that case, it would be better to allocate public resources where they are most needed. On the other hand, if a company is too far from an exporting status, one could question the utility of investing in that project and focus on how to reduce the distance from successful exporters. Note how credit scores with predictive models are nowadays common in the financial industry,when credit risk is measured by the probability that a debtor goes bankrupt. In our view, predicting success in foreign markets is apparently more challenging than predicting a firm’s failure. Yet, the prediction accuracy we obtain with the baseline predictive model is relatively high,as we make a mistake only once in every ten observations.
A second more sensitive case for which it is useful to know the next exporters are industrial policies for growth and diversification. After the recent global shocks, promoting diversification and developing new industrial capabilities have become a priority (IMF 2022) to tackle broader market failures, unfavourable business environments, and emerging sources of geopolitical risk. Over the last decade, the EU has elaborated a few general principles for the EU industrial policy (European Union 2023), which integrates into a number of EU-related policies, including external trade and the internal market. Against this background, it is useful to know where one can spot fringes of potential exporters, which could enhance the trade potential of a country, a region, or an industry. More technically, exporting scores detect ex-ante how trade extensive margins can change to catch how competitive regions or industries are becoming.
Let’s consider, for example, the map reproduced in Figure 2, where we can detect the geographic concentration of potential exporters in France whose odds of exporting are higher than the median company. Notably, we can briefly comment that there is more room for an increase in trade extensive margins in regions that are coloured green. Most notably, Paris and Île-de-France are not coloured in green, although we know that the capital region hosts many exporters. Instead, a grey area indicates that there is no significant concentration of exporters in that region. If we look better, a greater fringe of potential exporters sits in other areas of the country, although in places where the density of industrial activity may be lower. Eventually, fewer exporters are expected from the South of the country and overseas territories.
Figure 2 Where are the next exporters?
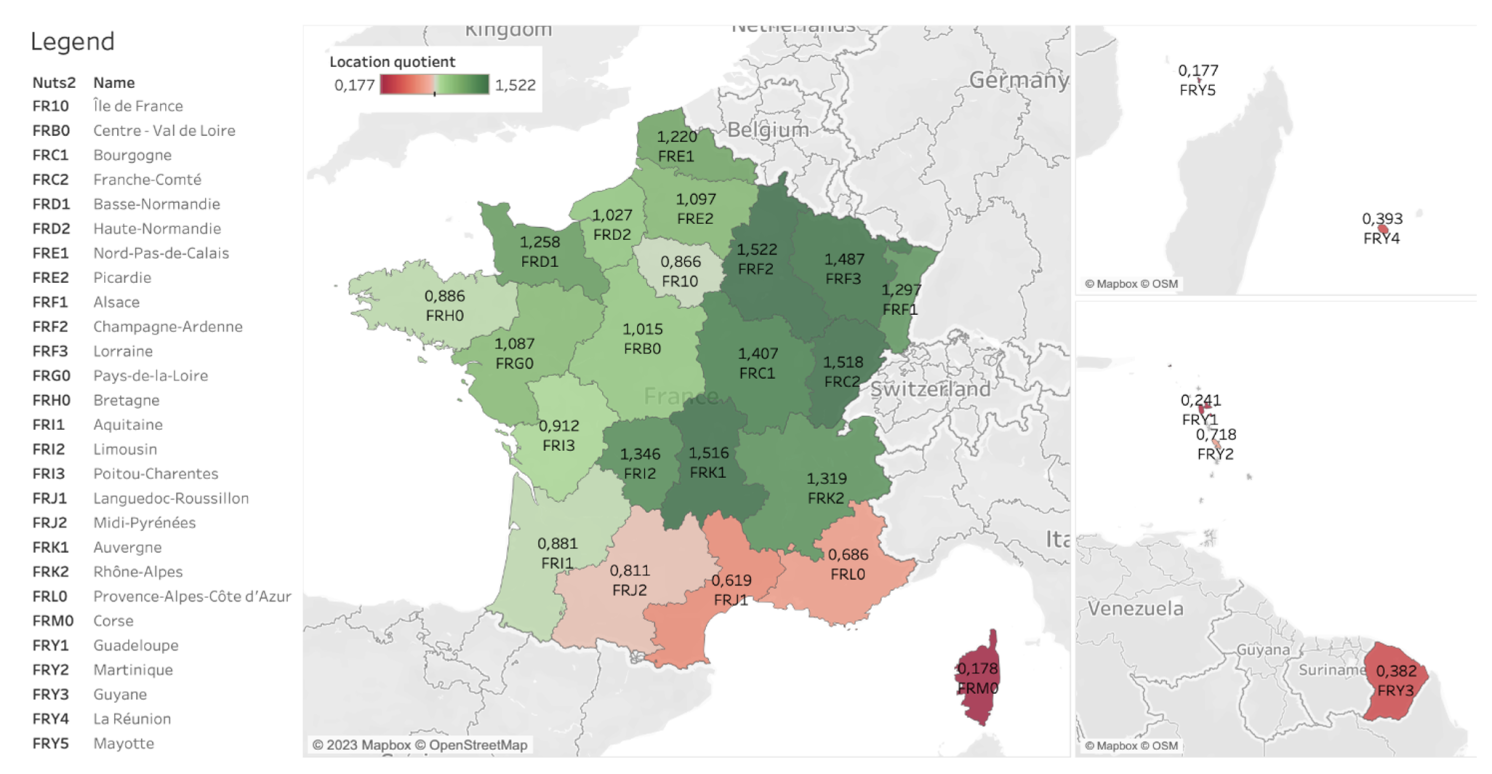
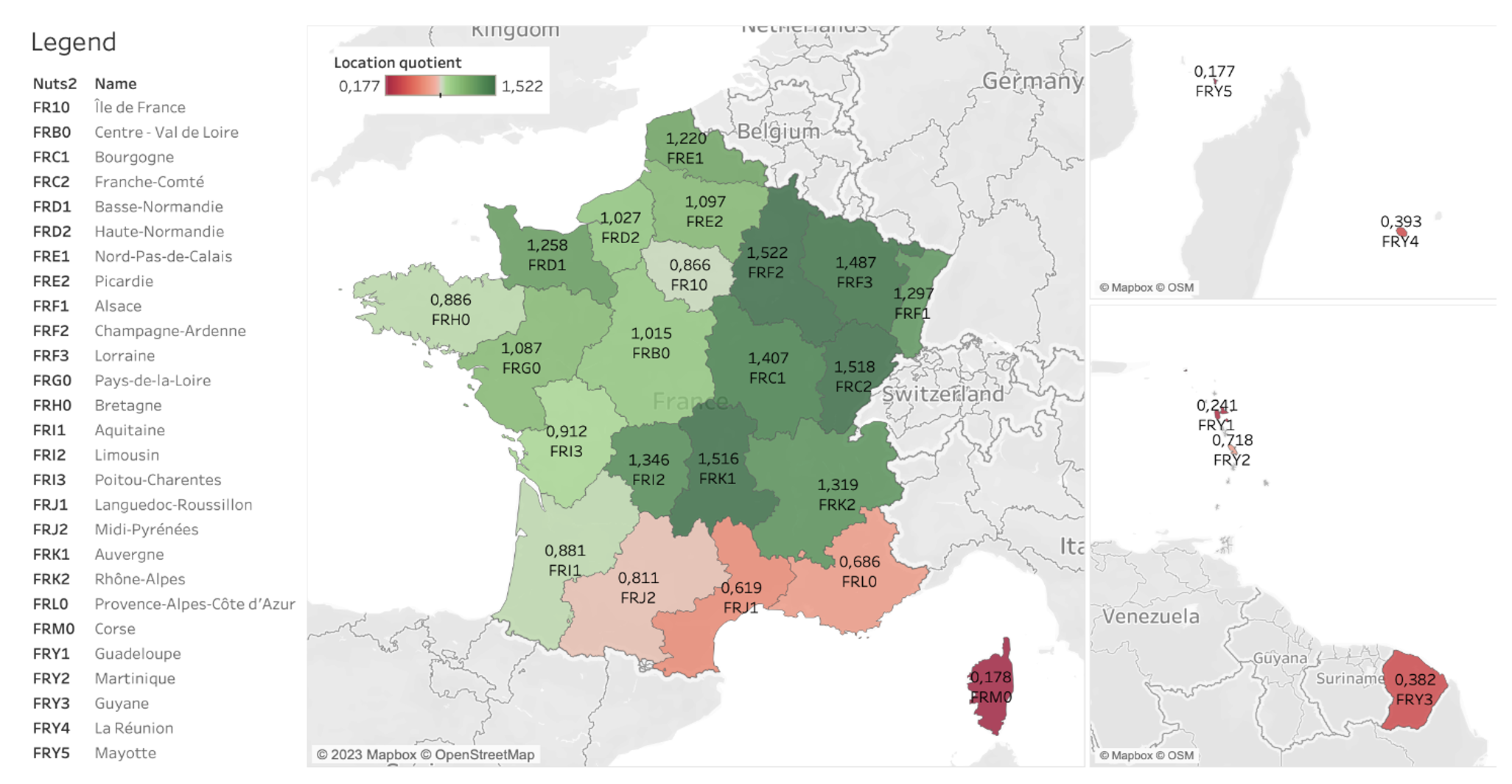
Note: Location quotients by NUTS 2-digit regions in France
Source: Micocci and Rungi (2023)
More clever analyses could investigate which firms in which industries are better positioned to become the next exporters, and one could also comment on the power of different indicators included in the battery of predictors. However, at this stage, we would not know how and why firms are in a condition to export. The latter is a relevant limitation of any ex-ante predictive model, which can only inform policymakers with a picture of the situation. Predictive models do not substitute structural economic models, policy evaluation methods or impact analyses. As in the case of modern macroeconometrics, forecasting gross outcome or inflation can be an essential exercise for a central banker, who however still needs to resort to other tools to design an optimal policy.
To conclude, modern data science has expanded the possibilities to make better, faster, and more efficient decisions based on empirical evidence. International trade scholars and policymakers can resort to machine learning predictive models and, thus, have more strings in their bow to design evidence-based policies. However, as the old-fashioned science fiction novel would remind us, we always need to take care of the Minority Report(s), i.e. when there is a minor, albeit non-negligible, chance that machine predictions may be wrong, and foreseen events can change by human intervention.
Source : VOXeu