Wage inequality is on the rise as much as wealth inequality. There is consensus that part of this rise is due to technological progress. The exact mechanism by which modern technology adoption affects inequality, however, is still open to debate. Katz and Murphy (1982) have popularised the view that, by assigning large premia to education, ‘skill-biased technological change’ (SBTC) raises wage inequality via vertical skill acquisition. Previous waves of technology improvements, however, did not produce the same effects, prompting researchers to ask what is special about the most recent waves. Recent studies indicate that the premium is not so much related to skill per se, but to the task content of each occupation. Certain tasks, particularly non-routine ones, are in high demand and earn extra premia, a view that has been labelled routine-task-biased technological change. While with skill-biased technological change new technology complements workers with high skills, with ‘routine-biased technological change’ (RBTC) new technology decreases demand for workers in traditional routine tasks while creating additional demand for workers in new complex tasks.
We push those ideas a step further by arguing that modern technologies such as automation and digitalisation increasingly require human operators with specialised knowledge of automated systems involving specific algorithms, software, and machines. The associated growing demand for specialised knowledge is conducive to a form of workers’ specialisation that increasingly matters above and beyond what would be needed by the high skill content of tasks or their routine intensity. This view implies that wage differentiation is due not only to vertical specialisation (higher knowledge more generally), but to horizontal specialisation (knowledge specialised to the task, no matter whether the latter is routine or complex). The underlying mechanism relies on the concept of positive assortativity of workers and firms/tasks: match surplus is the highest when the firm adopting the technology hires the worker specialised in that technology. If so, specialised workers’ wage also rise due to rent sharing arguments and so will wage dispersion (see also Benzell and Yifan Ye 2021 for the impact of automation on wage inequality).
To investigate this argument, we leverage a high quality confidential administrative dataset of the universe of contracts in the Italian system of industrial relations. The particular relevance of this type of data, relative to workers’ and firms’ surveys, is that it reports actual wages obtained from contracts of the universe of workers. To measure technology adoption, we use data from the International Federation of Robots (IFR) and construct a shift-share instrument which imputes the sectoral changes in the IFR stock of robots over value added to a local economy based on its sectoral employment shares.
The underlying hypothesis is that automation has increased assortativity, and hence specialisation, and through this it has increased the wage premium of specialised workers and the dispersion of wages. We test this hypothesis by devising an econometric strategy in two stages. The first stage measures the extent to which wages depend on assortativity, adopting and adapting recent econometric methodologies (specifically the finite mixture method by Bonhomme et al. 2019) aimed at precisely identifying and decomposing wages into a worker component, a firm component, and a third term given by the interaction of the other two components, which is meant to capture the complementarities between them.
Identification relies on workers who change employer: it is the same worker moving across firms who allows researchers to identify the firm premium. In other words, firms can be ranked in terms of their wage premia to the extent that they are ‘connected’ through at least one mover. Very often the fraction of movers is too small to allow for proper identification. The finite mixture methodology overcomes this hurdle through a pre-grouping procedure, by which similar firms are assigned to the same group and workers move between groups. In the second stage of our econometric strategy we use an instrumental variable approach to study the impact of robot adoption on the estimated complementarity (‘sorting’) between workers and firms.
In describing our findings, it is important to start from some facts that emerge from a simple statistical wage variance decomposition into between-firm and within-firm dispersion, which we entertain prior to the econometric analysis. This decomposition (see Figure 1) shows that the between-firm component is predominant, which may seem to suggest that mainly differences in firms’ characteristics explain the dispersion of wages. However, the variance analysis from the finite mixture methodology described above depicts a different picture (see Figure 2): differences in firms’ estimated characteristics explain much less of the wage dispersion as the contribution of workers’ estimated contribution is predominant and the contribution of complementarities between firms’ and workers’ characteristics is as important as that of firms’ characteristics.
Figure 1 Wage variance decomposition within and between firms


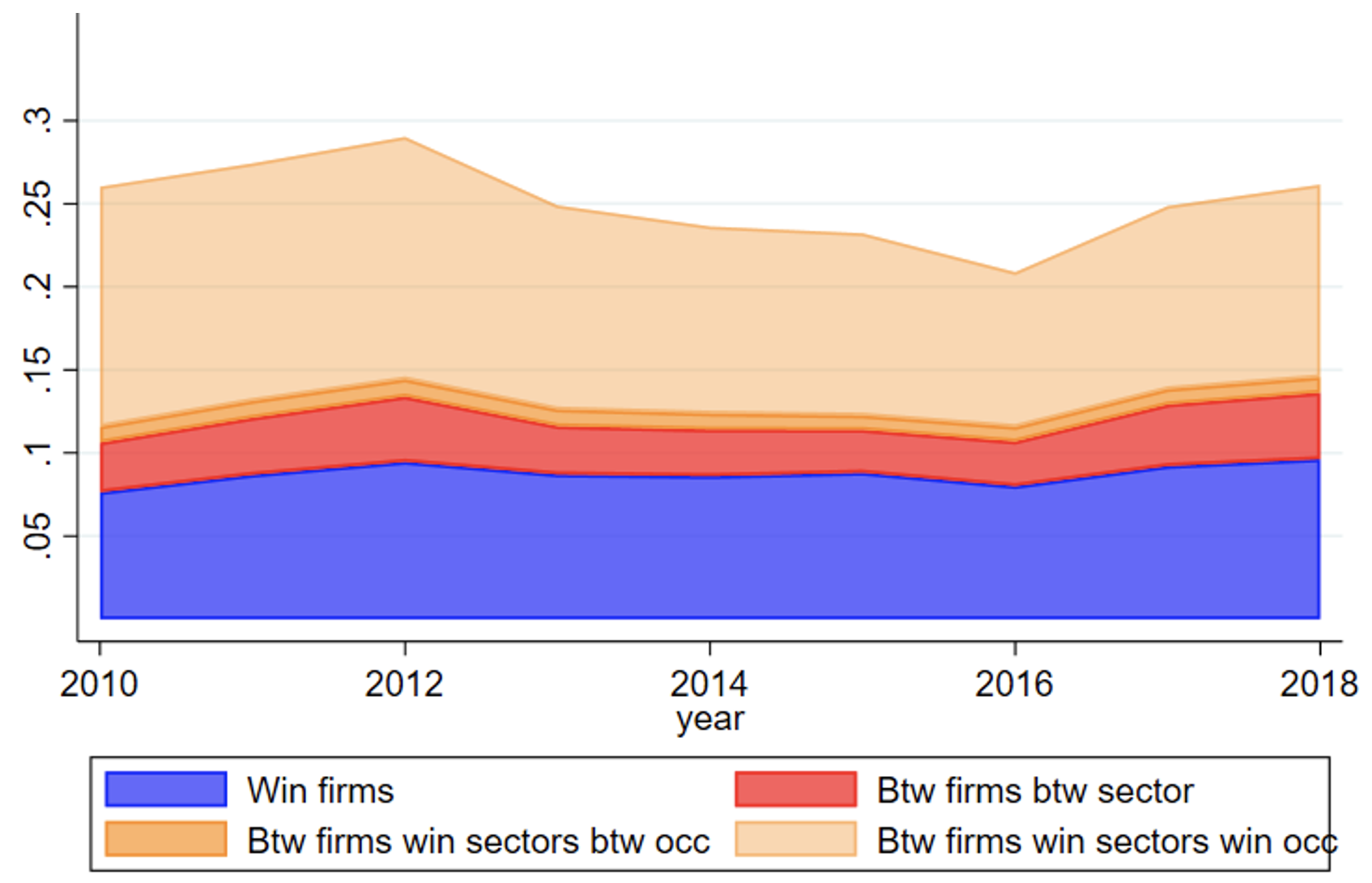
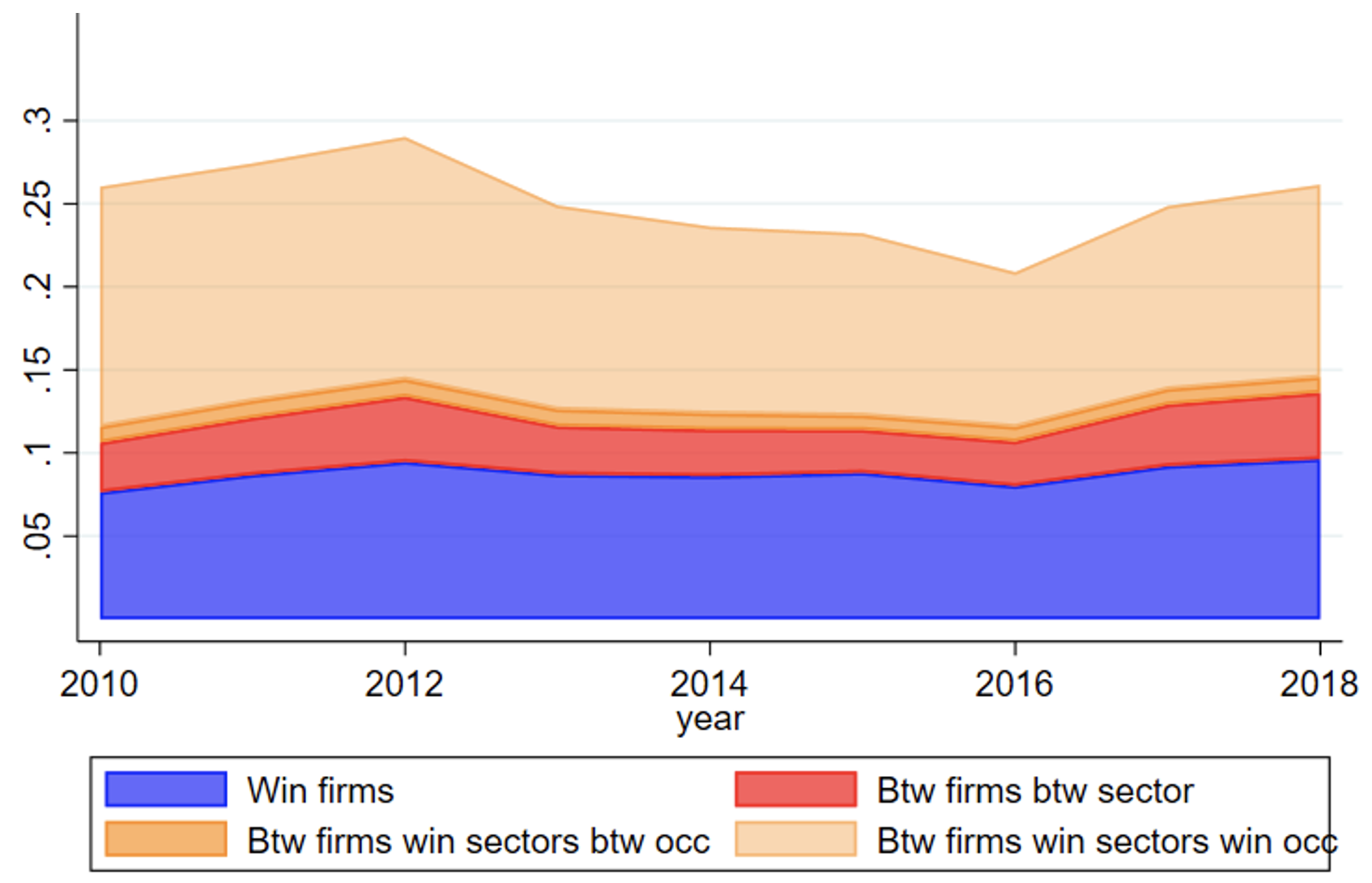
Notes: Wage variance decomposition in terms of four components: within firms (blue), between firms and between sectors (red), between firms, within sectors and between occupations (dark orange), between firms, within sectors and within occupations (light orange).
Figure 2 Wage variance decomposition: Finite mixture model
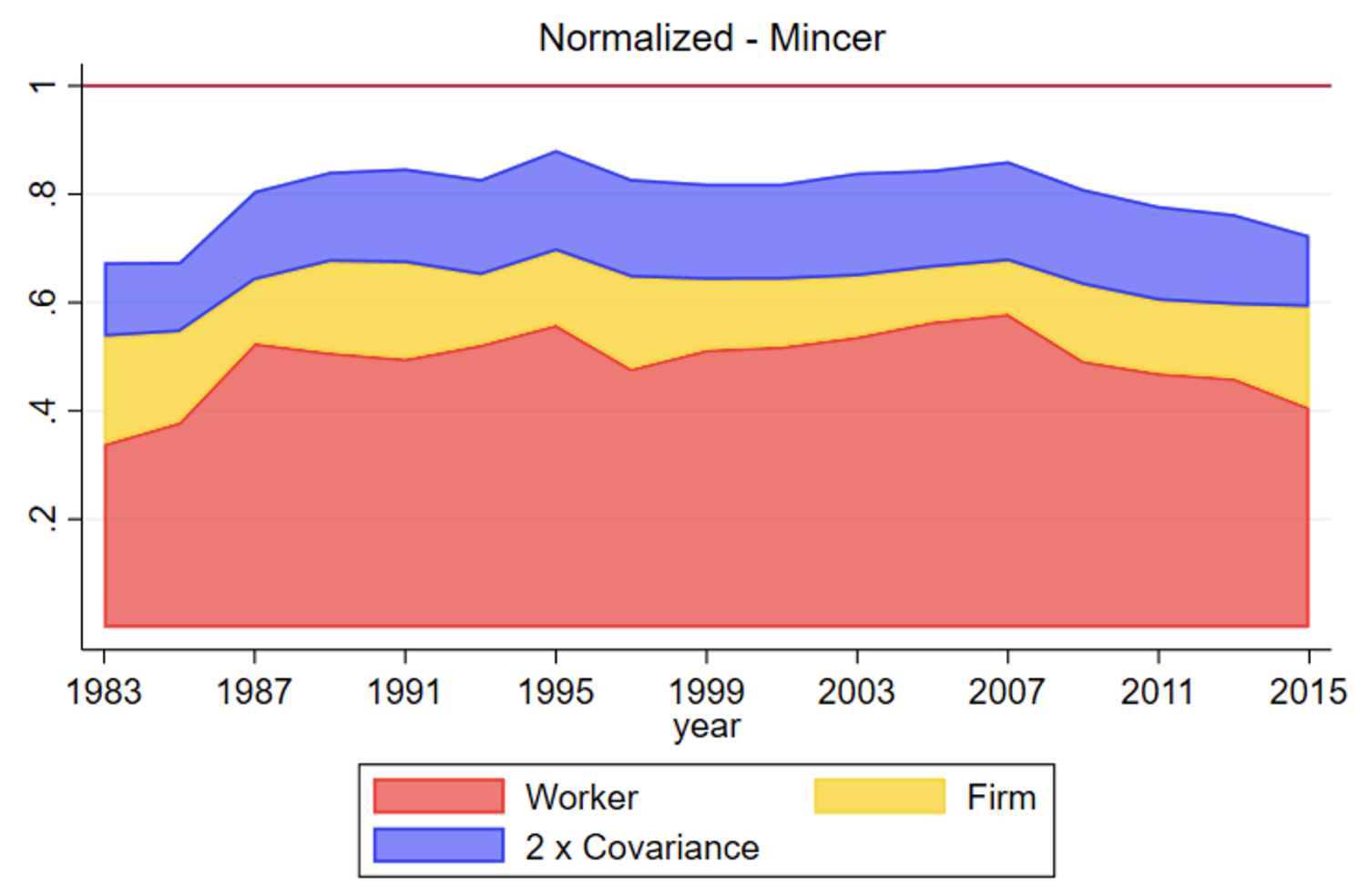
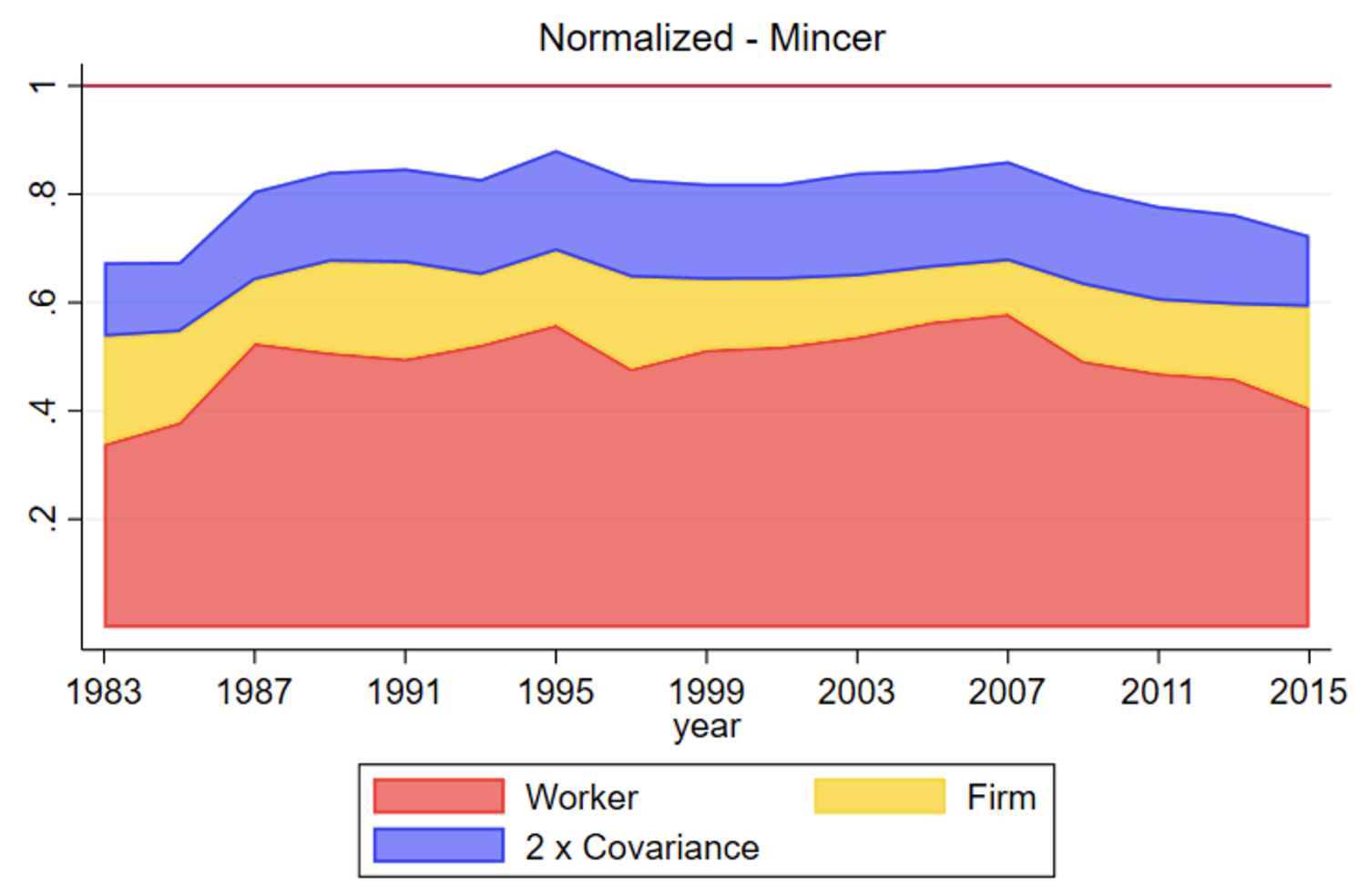
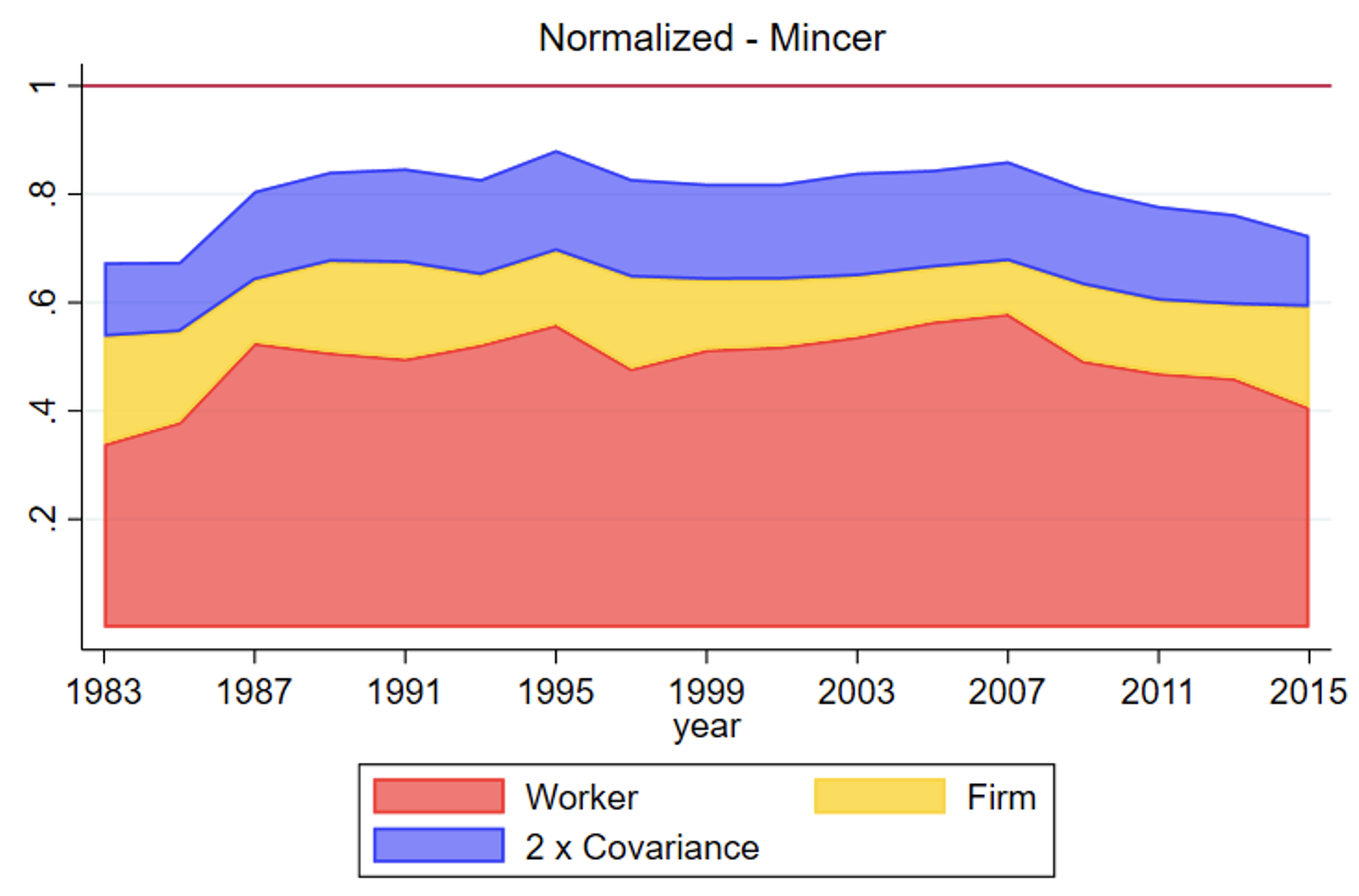
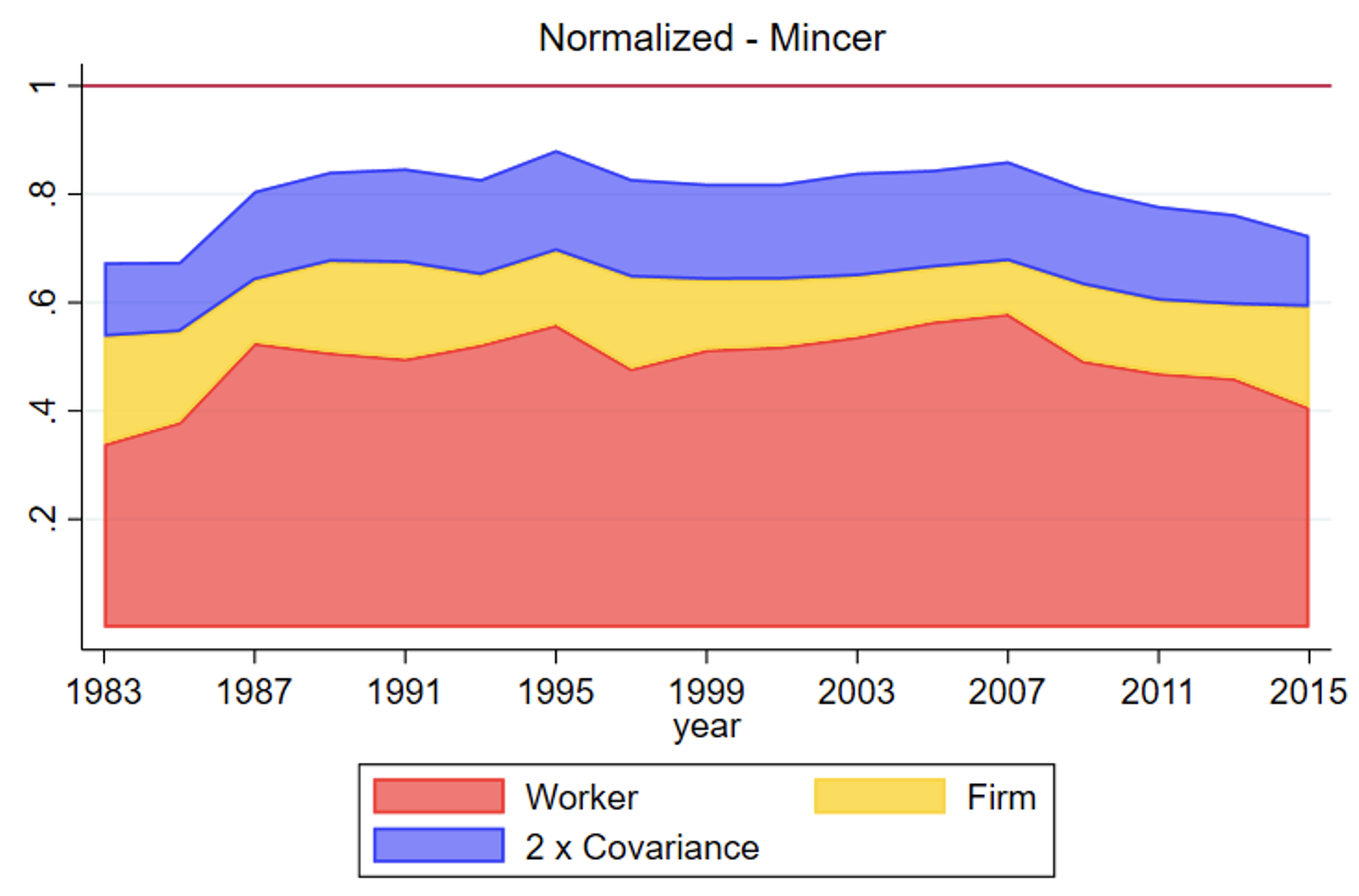
Notes: Wage variance decomposition in terms of estimated worker (red) and firm (yellow) unobserved characteristics and their correlation (blue).
In the second stage of the econometric methodology we link the estimated complementarity to our measure of robot adoption and find a significant and robust positive causal relation from the latter to the former, which holds across a battery of robustness checks: automation increases worker-firm sorting and increased sorting contributes to rising wage dispersion (see Steibale et al. 2020 for the role of robots for firms at the top of the distribution). We corroborate our results further by constructing a workers’ segregation index that measures concentration of similar workers in similar occupations and find that also segregation is positively affected by automation.
We show that our evidence can be explained within a class of models with two-sided heterogeneity, on-the-job search, rent sharing, and employee poaching, in which workers continuously search for firms that are more complementary to them than their current employers. In this framework, the impact of automation can be rationalised as strengthening the complementarities between workers’ specialised skills and firms’ specific tasks and disproportionately increasing the endogenous search intensity for workers with better characteristics (who stand to gain the most from better matched employers). Stronger complementarities and higher search intensity for better workers increase wage dispersion with a relevant role played by both between-firm and within-firm dispersion as seen in the data.
In sum, our work highlights that automation and digitalisation may not simply substitute workers with capital, but also enhance worker task complementarity in a way that exacerbates the between-firm dispersion of the wage distribution. It remains an open question what will be the welfare consequences and the growth prospect stemming from this mechanism.
This blog post is based on data from the recently published Voxeu.