Digital marketplaces have grown dramatically in recent decades, enabling buyers and sellers to trade goods and services, from hand-crafted products to short-term rentals. And though online platforms have disrupted traditional commerce, they have also replicated some of its biases. This column investigates the challenge for digital marketplaces seeking to both maximize revenue and prevent discrimination. By creating profile pictures that differ by race, ethnicity, gender, and social class – and estimating how different attributes impact lender demand – the authors evaluate various platform policies and identify which approaches best resolve the fairness-efficiency tension.
The last two decades have seen dramatic growth in digital marketplaces, where buyers and sellers connect to trade goods and services ranging from short-term rentals to rides to hand-crafted products to dog-walking.
Although digital marketplaces may change and even disrupt traditional commerce, they may just as easily replicate familiar biases from the physical world. There is a growing body of research that finds substantial inequities in outcomes between socio-demographic groups in many major online marketplaces. How can such factors play a role when most digital marketplaces match-up partners to a transaction before a face-to-face meeting occurs? In many marketplaces, participants have profile pictures that reveal their race, ethnicity, and gender, and may also provide clues about their social class.
Several recent papers attempt to isolate the effects of the demographics of buyers and sellers on marketplace outcomes. Edelman et al. (2017) conduct a field experiment on Airbnb using fake accounts and comparing the outcomes between accounts that are identical except for their names. They show that accounts with Black-sounding names are 16% less likely to have their rental requests approved than accounts with non-Black-sounding names. Farajallah et al. (2019) analysed a similar question in a study of the French ridesharing marketplace BlaBlaCar. Using transaction data, they showed that adjusting for the quality of the car, the age and gender of the driver, and the total supply of drivers on the specific route, drivers with Arabic-sounding names sell fewer seats than drivers with French-sounding names. In all of these cases, personal images in user profiles enable discrimination based on race or ethnicity. This empirical evidence has prompted proposals for platforms to reduce the prominence of personal images or even to remove them entirely (Fisman and Luca 2016 ).
However, profile images can also improve the efficiency of digital marketplaces and play a role in inducing good behaviour. Simply remembering that a human being exists on the other side of a transaction can affect behaviour. Some studies have attempted to quantify the impact of having a more trustworthy photo, as subjectively rated by observers. For example, using Airbnb data and a recruited experiment simulating the Airbnb experience, Ert et al. (2016) show that hosts with images labelled as ‘more trustworthy’ set higher prices and are selected more frequently.
In our study (Athey et al. 2022), we dig more deeply into the trade-offs between efficiency and equity that may arise in digital marketplaces, and examine different policies that platforms could plausibly implement around profile pictures. Marketplaces can make choices, for example, that encourage participants to create profile photos in a certain way. They can also make choices about how the pictures are employed in the marketplaces, using images as inputs into recommendation systems.
We analyse the impact of policies like this on efficiency and equity. We start by introducing a distinction between things that users can choose about their images, which we refer to as ‘style’ features, and intrinsic features of individuals, which we call ‘types’. Style features might include facial expressions, objects in the image, or image composition. This distinction is important because platforms might influence the style of participants’ profile pictures, for example by educating them about which styles are attractive to trading partners. It is also important for understanding the sources of inequities in marketplaces. Inequities might arise directly, when participants care about the types of their trading partners, but they may also arise indirectly, if one type of participant is more likely to make choices about style that other participants like. More precisely, when participants have preferences about style features, and styles and types are correlated, then the distribution of style features will impact the inequity between types.
We use this framework in the context of Kiva, an online microlending platform. Kiva matches lenders, typically impact-oriented private individuals, with borrowers, who are typically entrepreneurs from developing countries raising funds to advance their small businesses.
We begin our analysis by asking which characteristics of borrower profile pictures are predictive of getting loans quickly, and which characteristics are predictive of repaying the loan. To analyse features of images in a large-scale dataset, we deploy an off-the-shelf image-feature-detection algorithm that creates computer-generated features of images. Examples of features include whether the image is blurry or the subject is wearing glasses. Then, using our subjective judgment, we divide these features into two groups, features that relate to style and those that reflect types. We show that style features are important for predicting lender choices, but are not important for explaining the probability that the borrower will pay back the loan. In other words, there are differences in funding outcomes that are due to the style of borrowers’ profile images that cannot be accounted for by the borrowers’ underlying risk profile. These types of disparities can be understood as unfair because the funding outcome is unrelated to the creditworthiness of the proposed investment.
Next, we try to isolate the effect of each of the image features. Since features tend to be correlated with one another – that is, since image attributes tend to go together – it is important to compare apples-to-apples when interpreting the relationship between image characteristics and lender choices. This is difficult to accomplish because images have many different characteristics. Fortunately, machine-learning methods can be used in combination with techniques from the causal inference literature to adjust for many characteristics of images at once, and can in principle produce reliable estimates. Using these approaches, we estimate that smiling increases the amount of cash collected per day by approximately $8, while a body shot – in which the body of the borrower occupies a substantial part of the image – decreases the outcome by $10.
Figure 1 Pearson correlation coefficient between ‘style’ features in columns and ‘type’ features in rows
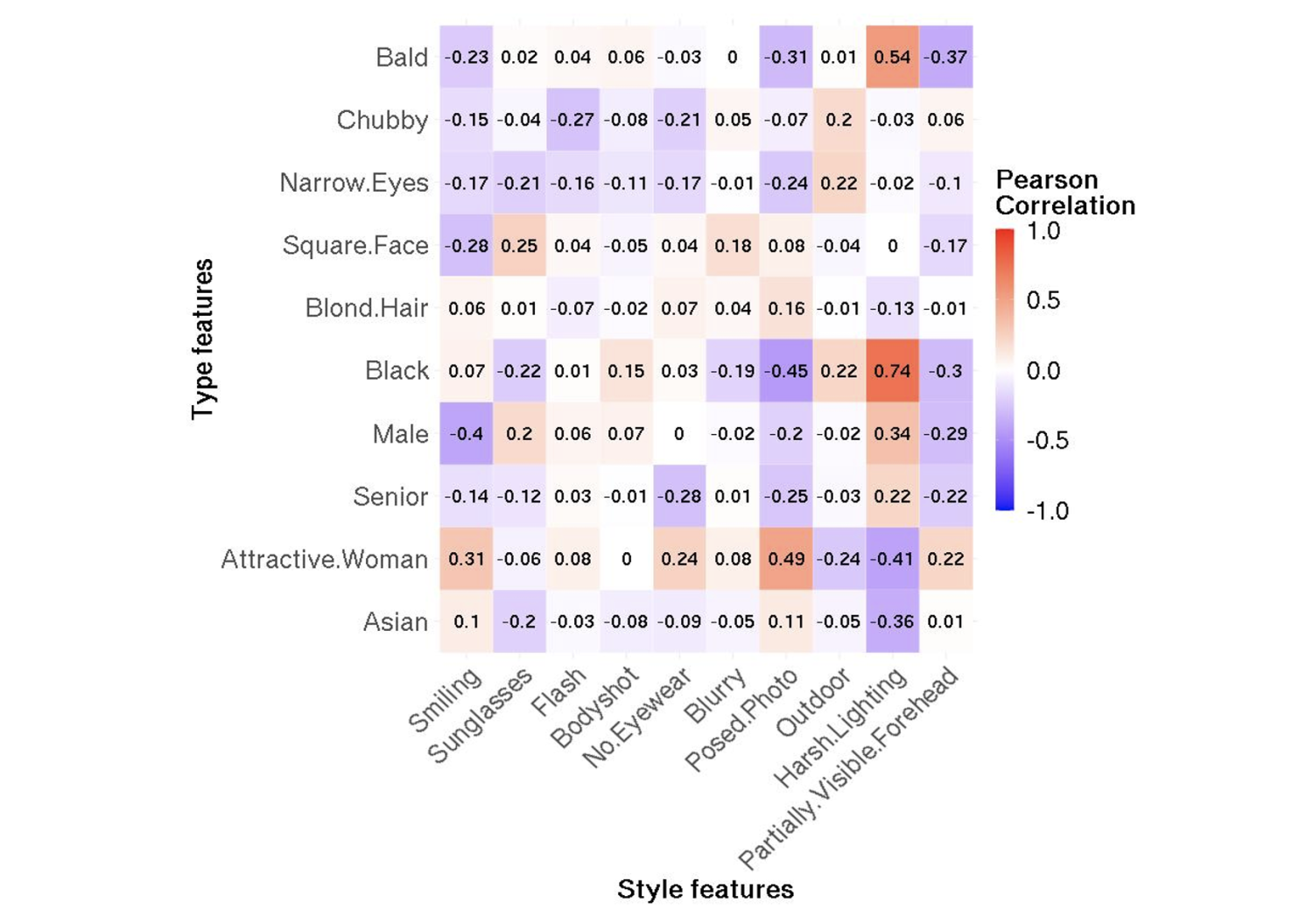

We also find systematic differences in the style of profile images across types. Figure 1 shows correlations of selected features. For example, 33% of profiles classified as male are also smiling, compared to 77% of profiles classified as female.
We quantify how the distribution of style features impacts the inequities between types using a covariate decomposition (Gelbach 2016). We find that differences in outcomes between several socio-demographic groups can be accounted for, to a large degree, by differences in style. For example, we estimate that the male-female funding gap would diminish by approximately a third if style features were equally distributed between males and females.
In this analysis of Kiva transaction data, our estimates of the impact of specific features in images on funding outcomes can be clearly interpreted only if we have adjusted for all other meaningful differences between profiles. Even though we use state-of-the-art feature detection algorithms, we cannot be certain that we have extracted all information from the images that would be relevant to lenders. To corroborate these results, we supplemented our analysis of real-world Kiva transactions with an experiment conducted with recruited subjects, in which we are more confident, by design, that the images differ only in one dimension.
We focus on two style features: smiling and body shot. Based on the analysis of Kiva data, we know that these features are uncorrelated with repayment probability, and they contribute to inequities between several types. To study the impact of the specific image features, we generated images that differ only in one feature at a time. To do this, we made use of a machine-learning algorithm known as Generative Adversarial Networks, or GANs (Goodfellow et al. 2020).
We generated eight variants of each image and used them to create borrower profiles (see Figure 2 for an example). Subjects in the experiment are randomly assigned to a variant and asked to choose between two profiles. We estimate that subjects were more likely to choose borrowers who identify as female (an increase of 31%) and borrowers who smile (an increase of 34%), and less likely to choose borrowers with profile images that are body shots (a decrease of 17%).
Figure 2 Two variants of the same image
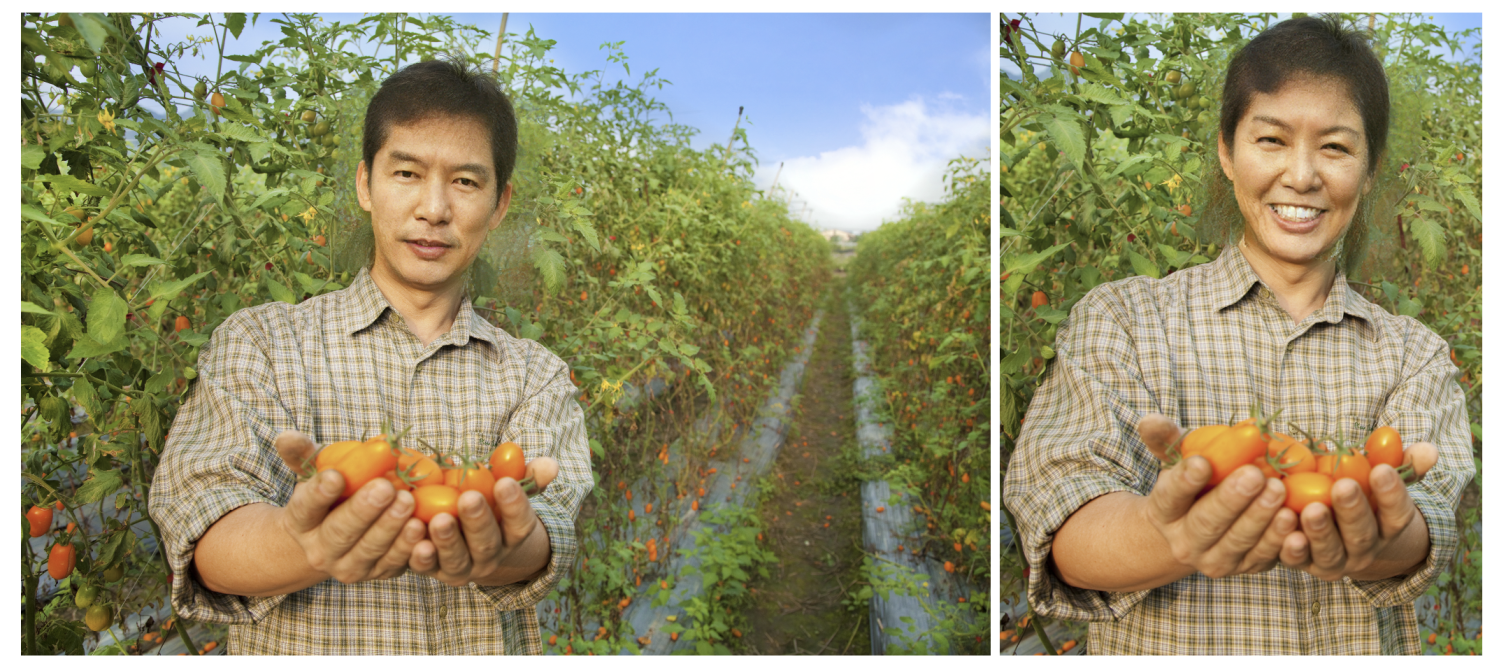
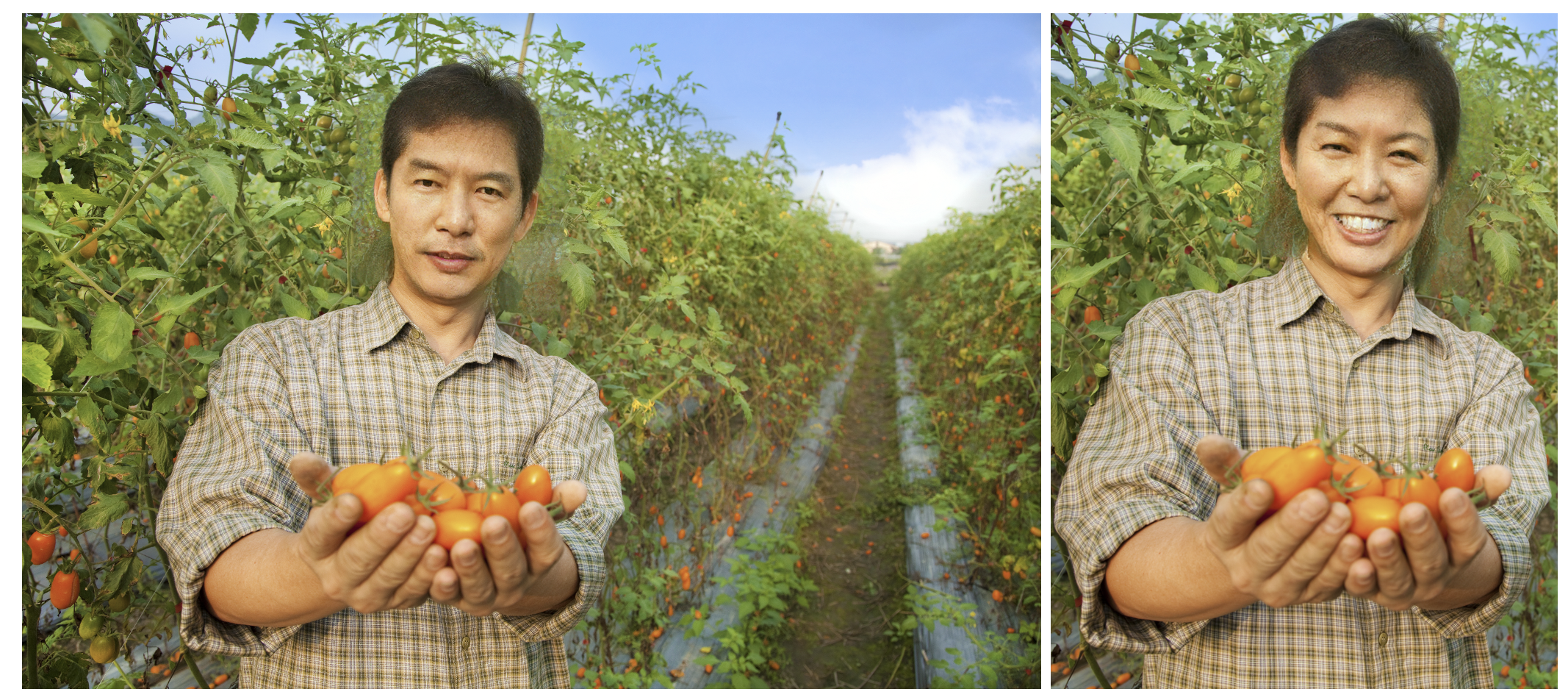
Note: Left variant: male, not smile, not body shot. Right variant: female, smile, body shot.
Our results show that styles of profile images contribute to inequities in outcomes across socio-demographic groups. However, marketplaces don’t need to take the style of user profiles as given. Instead, the style features can be altered by users; in particular, the platform can encourage users to make different choices in their images.
We propose several potential policies that marketplaces might consider. In particular, we develop a simple model wherein behaviour is linked to the estimates from our empirical work. In our model, lenders have preferences with regard to types and styles, and choose a borrower to maximise their utility; they can also decide not to lend money on the platform. The platform impacts outcomes in two ways: through the choice of borrowers it presents to lenders (we assume that the platform observes a pool of borrowers and selects a subset), and in whether it nudges borrowers to change their style. Platform decisions impact efficiency, which we measure by the share of lenders that choose one of the borrowers instead of the outside option; and fairness, as captured for example by the Gini coefficient of the distribution of funds.
In our model, policies that change which borrowers are shown to lenders can either improve fairness or efficiency, but never both. For example, a policy of recommending profiles with desirable style features increases efficiency but is detrimental to fairness. By promoting profiles with desirable features, the platform promotes types that achieve high outcomes regardless, increasing inequity.
However, there is a class of policies that can increase both efficiency and fairness. Consider a policy in which the platform recommends or nudges non-compliant borrowers to create profiles with desirable style features, and that both high- and low-performing types comply to a similar degree. This reduces the correlation between types and styles, which improves fairness, and efficiency is higher when there are more profiles with attractive features.
As online marketplaces become more important, the economic costs of inequities in outcomes for users will grow as well. In Athey et al. (2022), we identify platform policies that influence users to change specific aspects of profile images, leading to more equitable outcomes between socio-demographic groups while simultaneously increasing the efficiency of marketplaces.
Source : VOXEu